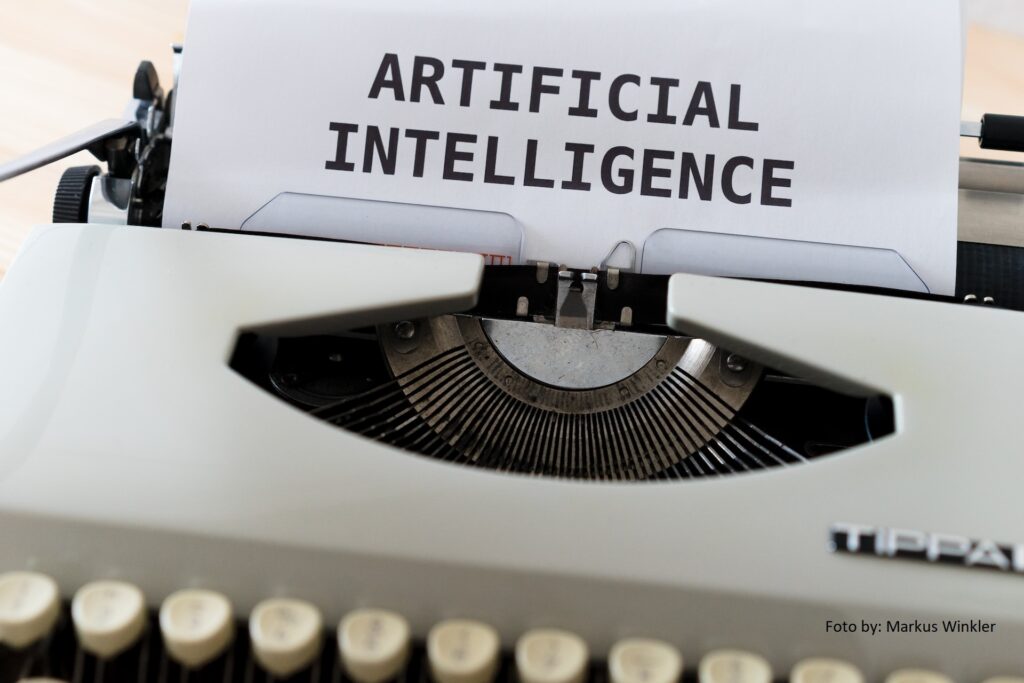
In the spring of 2023, I had the opportunity to participate in a Master Class on Artificial Intelligence (AI) and the derived ethical and legal challenges organized by the University of Copenhagen and Copenhagen Fintech. Since then, I have reflected on the consequences AI will have for us project managers when we lead AI projects.
The management of AI projects poses several unique challenges due to the interdisciplinary nature of these projects, involving both technical and non-technical aspects. In the following, I will outline some of the key project management challenges related to AI projects.
AI projects often involve complex problem-solving, and the project’s initial scope can be ambiguous and change over the course of the project; therefore, defining clear goals and requirements will be challenging, which can cause difficulties in project planning and execution. Therefore, the traditional waterfall model often has to give up when working with AI, and we must choose alternative paths.
AI models largely depend on the quality and availability of data. It can be a significant challenge to gain access to all relevant and sufficient data, to clean and pre-process it and to handle it correctly concerning, for example, data protection and never to be forgotten the GDPR legislation.
The interpretation and explainability of AI models are crucial, especially in regulated industries such as insurance and banking or in the handling of sensitive information. Ensuring that AI models can be understood and explained will pose a significant challenge in many projects. Not least when the legal requirements for the use of AI are tightened, as is the case with the upcoming EU legislation, where the use of artificial intelligence in the EU will be regulated by the AI Act, the world’s first comprehensive AI law. In addition, the AI field is developing rapidly with new algorithms, frameworks and tools. Keeping up with the changing technology landscape can be challenging and may require continuous learning and adaptation during the project.
Another critical aspect is that AI models can inadvertently perpetuate the biases found in the training data, which can lead to ethical concerns and risks. Addressing biases, ensuring fairness, and addressing ethical considerations are important points of attention that an AI project manager must learn to address. According to the upcoming EU legislation, there are several unacceptable risks that an AI project must deal with because they pose a threat to humans and will, therefore, be banned. These risks include:
- Cognitive-behavioural manipulation of people or specific vulnerable groups: for example, voice-activated toys that encourage dangerous behaviour in children.
- Social scoring, such as classifying people based on behaviour, socioeconomic status, or personal characteristics.
- Biometric identification and categorisation of persons
- Real-time and remote biometric identification systems, such as facial recognition
Some exceptions may be allowed due to law enforcement. Real-time remote biometric identification systems will be allowed to a limited extent in serious cases. In contrast, remote biometric identification systems, where identification is carried out with a significant delay, will be allowed to exist for the prosecution of serious crimes and only after approval by the court.
The interaction between AI solutions and existing systems and workflows can be challenging and is clearly a risk. Compatibility issues, inconsistencies in data formats, and the need for seamless integration can pose significant difficulties in deployment.
In AI projects, we often have a longer development cycle compared to traditional software projects. The iterative nature of model training, testing, and improvement can lengthen the project timeline, making it challenging to deliver results within the expected timeframe.
Therefore, it will also be a challenge to assess the return on investment (ROI) of AI projects when the project’s success metrics are not well defined. Establishing clear key performance indicators (KPIs) and measuring the added value of the IT solution is crucial for most projects, but for AI projects, they can be completely uncertain.
AI projects often require collaboration between data scientists and domain experts. The absence of domain expertise can lead to misunderstandings, misalignment of goals, and lack of context, affecting the effectiveness of the AI solution. For example, suppose you want to try to streamline the processes in an insurance company. In that case, you must know the need for domain knowledge concerning the content of insurance claims and the legal-driven requirements.
In general, onboarding and retaining the right skills is an essential prerequisite for success because, as mentioned, AI projects often require specialized skills, including computer science, machine learning, and domain-specific expertise. Acquiring and retaining qualified personnel can, therefore, be a significant challenge, leading to resource constraints and potential delays.
Addressing these challenges requires a well-structured project management approach that includes flexibility, collaboration between technical and non-technical teams and, not least, ongoing communication with stakeholders and the project steering committee. Regular risk assessments and a focus on mitigating potential problems throughout the project lifecycle are thus extremely important when it comes to AI projects.